Data is everything. It drives product decisions, investment choices, what customers to reach out to and when in their lifecycle… it can even raise early flags about an organization’s health. The question is, are you fully realizing the value and potential of your data?
A data governance framework allows you to maximize the insights around the data you collect, store, and create and reduces your risk for issues from backup lapses to security breaches. If your organization needs to adhere to any compliance requirements or needs to develop a competitive advantage, data governance is the answer to giving you that edge.
With a data governance framework, you can gain the accountability and transparency you need to improve operations and standardization throughout your organization. And maximized efficiency means higher profit margins, less room for error, and less resource bleed. Follow these eight steps to move from the data brainstorming stage into a place of action.
1. Define your data governance objectives and project scope
In defining your data governance plan, start by clarifying your objectives and scope. What are your primary goals? For example, is it to improve data quality, streamline compliance requirements, and improve organizational security? Maybe you are looking to create a cleaner line between data findings and decision-making. Write out what your goals are. This data governance ebook is a great resource for common data governance goals and for navigating the scopes and objectives phase of your data governance plan.
2. Establish the Roles and Structure for your Data Governance Plan
Many organizations opt to have a data governance committee made up of representatives from different departments including IT, legal, compliance, customer success, and sales. Anyone touching the data should be involved or aware of the data governance plan. A data governance committee functions to give oversight and strategic direction for the data governance program. The committee is responsible for defining and approving data governance policies, standards, and procedures. Clearly defined roles and responsibilities within the committee help create oversight and accountability for data processes.
Here’s an example of what the roles within a data governance committee might look like but it could vary depending on your organization’s size, industry, and specific goals.
Role | Responsibilities |
Data Steward | Ensure the quality, integrity, and security of specific data domains or datasets. – Define and enforce data standards and best practices within their domain. |
Data Owner | Define data policies, access controls, and usage guidelines for specific datasets or data assets. – Ensure activities are compliant with regulatory requirements. |
Data Custodian | Implement technical solutions and enforce data governance policies and controls. – Manage data storage, access controls, and encryption mechanisms. |
Data Governance Officer | Lead the data governance program’s development, implementation, and enforcement. – Define and communicate data governance policies, procedures, and standards. |
Who is responsible?
The way your data governance model distributes responsibility of data governance will depend on factors such as organizational culture, size, complexity, regulatory environment, and strategic objectives, but ultimately every employee is responsible for following best practices with data privacy and storage as it applies to their role within the organization. Here are a few different governance models your could use when building your data governance committee to structure who is responsible for setting these policies.
Data Governance Responsibilities | Description |
Centralized | Involves a central governing body or committee responsible for decision-making, policy-setting, and oversight of data governance activities across the organization. |
Decentralized | Distributes data governance responsibilities across different departments or business units, allowing each to develop its governance processes and policies. |
Hybrid | Combines elements of centralized and decentralized approaches, offering central oversight while granting some autonomy to individual departments or business units. |
Federated | Shares data governance responsibilities among multiple entities such as business units, divisions, or subsidiaries, each having its governance structure. |
Cooperative | Emphasizes collaboration and shared responsibility among stakeholders from different departments or organizations, promoting cross-functional engagement and consensus-building. |
Regulatory-driven | Focuses primarily on compliance with regulatory requirements and industry standards governing data management and privacy, prioritizing risk management and legal compliance. |
3. Develop policies and standards
Once the data governance committee is set, and your objectives and scopes are agreed upon, it’s time to develop the policies and standards that will shape your data collection, management, and disposal. These policies, once formalized, are the bones of your data governance plan. Your policies, procedures, and standards are what guide successful data governance.
Data policy areas to consider defining:
- Data Collection Policy: Outline methods, purposes, and consent mechanisms for data collection activities.
- Data Storage Policy: Specify storage infrastructure, access controls, and encryption standards.
- Data Access and Sharing Policy: Define roles, permissions, and protocols for data access and sharing.
- Data Usage Policy: Provide guidelines for appropriate data handling, usage, and consequences for policy violations.
- Data Retention and Disposal Policy: Establish retention periods, archival procedures, and disposal protocols.
Data standards to consider defining:
- Data Naming Conventions: Ensure consistent and clear naming conventions for data elements.
- Data Formatting Standards: Define formats, encoding schemes, and delimiters for data interoperability.
- Data Classification Scheme: Categorize data based on sensitivity, criticality, and compliance requirements.
- Data Documentation Standards: Document metadata, lineage, and quality metrics for comprehensive data understanding.
- Data Integration Standards: Specify integration patterns, mapping rules, and transformation logic.
4. Assess current state
Now that you know what you want, it’s time to pause and measure your current state against the objectives and policies that you’ve identified. Conduct a thorough assessment of existing data assets, quality, compliance, and security posture to inform governance initiatives effectively.
A data inventory should include classifying your data types, and assessing these questions:
- Where is it stored?
- How is it used?
- Who uses it?
Create a workflow for periodic data assessments to help you determine what data to archive, retire, or identify risks for data ex: unpatched tools and programs that could be vulnerable to security holes.
5. Build a data model
Select a governance model aligned with organizational needs. Data models can be represented conceptually, physically, or logically.
A popular form of data modeling is called a data entity relationship diagram, or an ERD. It documents all of the data within your organization and the relationship between the different data using different icons and terms to represent data including, descriptions, values, constraints, and rules.
Here’s a a simple supply chain example we found on EDRAW.com
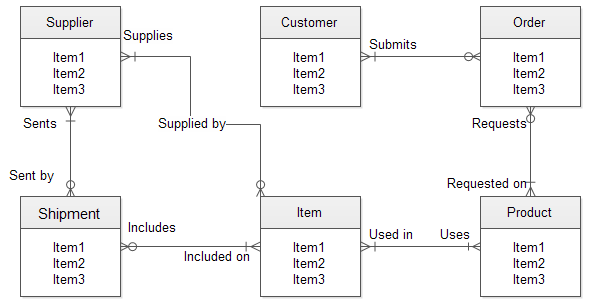
Data modeling is strongly correlated to a quality data governance program because it makes it easier to document data relationships, improves transparency where data problems occur, and makes complying with regulations easier to prove and maintain. Data modeling helps also create clear easy-to-understand data rules which can help with training employees on data discipline and meeting compliance requirements.
6. Implement data access controls
In addition to data classification and modeling and your lifecycle management workflows, access controls are important to put into place. In general, limited access to files, documents, and customer data to only those who need it to do their jobs is a rule of thumb. This is called the principle of least privilege or POLP. Each employee’s access to different tools, systems, and CRM visibility needs to be documented in a spreadsheet. Consider giving temporary or timed access for a few months if an employee needs access to something for a specific project that has a completion date. Have a kickoff call and make clear the before and after times so poor data management practices don’t continue to leak in from different areas.
7. Provide training and awareness
Offer training programs and awareness campaigns to foster a culture of data stewardship, compliance, and accountability across the organization. Have a kickoff call or make your data governance plan a headline in the quarterly company meeting. It’s critical to draw a line between the before times and after times with deadlines for any license expirations, changes in permissions, or expected behavior changes. Adding mandatory training for employees who violate the data governance plan helps keep poor data governance practices from becoming a security liability.
8. Monitor and measure performance over time
Finally, how do you know if your data governance plan has been a success? Define Key Performance Indicators (KPIs) to track and show the effectiveness of your plan over time. Here’s a table with example KPIs you might want to track:
KPI | Description |
Data Quality Index | Measure the overall quality of your data by tracking metrics such as accuracy, completeness, and consistency. |
Compliance Adherence Rate | Monitor the organization’s adherence to data protection regulations and internal compliance policies. |
Incident Response Time | Measure the time to detect, assess, and respond to data security incidents or breaches. |
Data Access Control Effectiveness | Assess the effectiveness of access controls by monitoring unauthorized access attempts and successful breaches. |
Data Usage Patterns | Analyze data usage patterns to identify trends, anomalies, and potential misuse of data assets. |
Data Governance Training Completion Rate | Track the percentage of employees who have completed data governance training programs and workshops. |
Data Lifecycle Management Efficiency | Measure the efficiency of data lifecycle management processes, including data creation, usage, storage, archival, and disposal. |
Data Governance Policy Compliance Rate | Monitor the organization’s adherence to data governance policies, procedures, and standards. |
User Satisfaction Surveys | Gather feedback from stakeholders to assess their satisfaction with data governance processes, tools, and support services. |
By tracking these KPIs regularly and analyzing trends, organizations can what is working in their data governance program, identify areas for improvement, and make the changes needed to continuously improve.
Conclusion
Follow these steps, and you’ll be well on your way to a working data governance framework. A data governance plan safeguards your sensitive customer data and enables you to use data to back important organizational decisions, stay compliant with regulations, and improve customer experience. Having a grasp on your data allows you to prepare for and respond quickly to whatever lies ahead, whether it’s the transition to a cookieless future, embracing new advancements in AI models, or adapting swiftly to regulation changes, or economic swings.